Expanding Agile Practices and Embracing Data Governance for Modern Organizations
Why change a running system? In a rapidly evolving digital landscape, embracing new methodologies and exploring broader perspectives becomes essential. Agile practices are more than just Scrum; they encompass a wide array of approaches aimed at optimizing organizational workflows. This journey led us to become certified trainers in Disciplined Agile and integrate this fresh knowledge into our projects. However, staying agile also means continuously seeking new methodologies and frameworks, like Data Mesh, that align with modern data needs.
In this article:
Adapting Agile Principles for Data Governance and Data Mesh
Our journey has taken us deeper into the realms of data architecture, governance, and the intersection with GDPR and organizational needs. Integrating data governance within an agile framework ensures a structured yet adaptable approach that allows innovation while maintaining control and data security. This shift promotes better domain ownership, federated governance, and viewing data as a product.
Key Components for Effective Data Mesh Implementation
- Standardized DevOps: Unified processes and seamless integration of tools to facilitate automation and consistency.
- Data Catalogue: A centralized source for metadata, data lineage, and ownership information to enhance transparency and usability.
- Federated Governance: Collaborative frameworks where domain leaders establish platform rules and sharing protocols.
- Governed Platform: A managed platform that supports efficient data sharing and collaboration across teams.
- Automation: Streamlined data provisioning, especially in the Data Lake and Data Vault, to avoid delivery bottlenecks.
- Release Management: Organized release notes to communicate new data products and functionalities effectively.
- Standard Guides: Comprehensive guidelines to ensure consistent data handling throughout the organization.
Why Data Governance Matters
Data governance should be a central focus for modern organizations. Research shows that only 11% of companies have a robust data governance structure, yet those that do experience significant benefits:
- Improved Efficiency: Effective governance can reduce data search time by up to 50% (IBM).
- Enhanced Decision-Making: Strong governance leads to 40% faster decision-making due to better data access (Databricks).
- Long-term Value: By 2027, 60% of companies may not realize their AI project potential due to inadequate governance (Gartner).
Core Elements of Data Governance
To establish an effective governance framework, focus on:
- Ownership: Clear roles for data stewardship and accountability for data lifecycle management.
- Accessibility: Authorized, user-friendly data access for stakeholders.
- Security: Robust data protection policies including encryption and access control.
- Quality: Continuous monitoring and improvement of data accuracy, completeness, and consistency.
- Transparency: Comprehensive documentation and metadata management to foster data literacy.
By integrating these agile and data governance principles, organizations can unlock true potential, fostering both innovation and compliance.
Watch the Video
Meet the Speakers
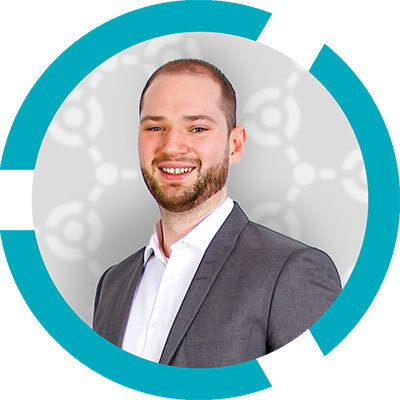
Lennart Busche
Consultant
Lennart is working in Business Intelligence and Enterprise Data Warehousing (EDW), supporting Scalefree International since the beginning of 2023 as a BI Consultant. Prior to Scalefree, he had over eight years of experience in the financial IT sector with focus on project management, IT-Service management and client management. This helped him get a broad knowledge of business requirements, the needs of customers dealing with IT and communication with different customer groups.

Lorenz Kindling
Senior Consultant
Lorenz is working in Business Intelligence and Enterprise Data Warehousing (EDW) with a focus on data warehouse automation and Data Vault modeling. Since 2021, he has been advising renowned companies in various industries for Scalefree International. Prior to Scalefree, he also worked as a consultant in the field of data analytics. This allowed him to gain a comprehensive overview of data warehousing projects and common issues that arise.