DBT Snapshots Question
This topic was inspired by a viewer’s question: “How can you build a Data Vault data warehouse downstream from dbt snapshots?”
In this article:
What is a Snapshot in dbt?
A snapshot in dbt is a tool that captures and preserves changes in data for tables that may be updated over time. This enables historical analysis and ensures that changes in data are properly recorded.
Key Features of dbt Snapshots
- Definition: Snapshots capture historical data by preserving changes over time.
- Purpose: They allow analysts to look back at previous versions of data, supporting historical analysis, auditing, and compliance.
- Mechanism: Snapshots are built on Slowly Changing Dimensions (SCD) Type 2.
- Value: Essential for auditing, regulatory compliance, and data analysis.
To create snapshots, use the command:
dbt snapshot
They are also included in the broader dbt build
command. Notably, snapshots cannot be rebuilt in a direct way to prevent accidental loss of historical data.
Metadata Fields
dbt adds four key metadata fields to snapshot tables:
dbt_scd_id
: Unique identifier for change tracking.dbt_updated_at
: Timestamp of the latest update.dbt_valid_from
: Start timestamp of the record.dbt_valid_to
: End timestamp (when applicable).
Starting with dbt version 1.9, these field names are customizable.
Snapshot Strategies in dbt
- Timestamp Strategy: Tracks changes using an
updated_at
column. Recommended due to its performance. - Check Strategy: Compares column values for changes. Useful when there is no reliable timestamp column but can be less efficient.
Handling Hard Deletes
By default, dbt does not track hard deletes. However, enabling invalidate_hard_deletes: true
ensures that deleted records are marked with an updated dbt_valid_to
timestamp. This is crucial for effectivity satellites in Data Vault modeling.
Demo: Data Vault Integration with dbt Snapshots
Source Data: Snowflake Sample Data
For the demo, we use Snowflake’s TPCH sample dataset:
- Database:
snowflake_sample_data
- Schema:
tpch_sf1
- Selected Tables:
customer
,orders
,part
,lineitem
Each table has a corresponding dbt snapshot:
snap_customer
snap_orders
snap_part
snap_lineitem
The snapshot configurations:
- Strategy: Check (due to lack of reliable timestamp columns)
- Hard Deletes: Enabled (
invalidate_hard_deletes: true
)
Target: Raw Data Vault Model
The Data Vault model consists of:
- Hubs:
customer_h
,order_h
,part_h
- Links:
orders_l
(customer-order relationship),orders_parts_l
(order line items) - Satellites:
customer_s
,order_s
,part_s
,orders_parts_s
- Effectivity Satellites: Track historical changes with
dbt_valid_from
anddbt_valid_to
During the demo, we focus on the customer entity, tracking how changes in the customer
table are reflected in its corresponding Data Vault satellites.
Conclusion
Using dbt snapshots as a persistent staging area (PSA) for a Data Vault-powered EDW enables accurate historical tracking. The combination of snapshot strategies and effectivity satellites ensures that data lineage and changes are well-documented and auditable. By leveraging Snowflake’s sample datasets, we can efficiently test and refine Data Vault implementations.
Watch the Video
Meet the Speaker
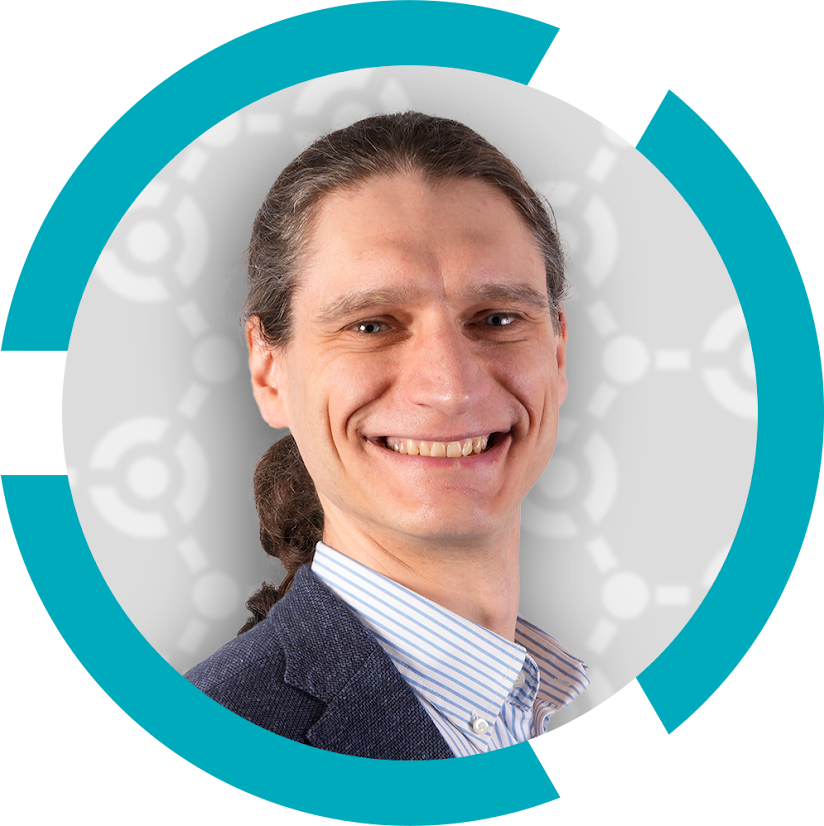
Dmytro Polishchuk
Senior BI Consultant
Dmytro Polishchuk has 7 years of experience in business intelligence and works as a Senior BI Consultant for Scalefree. Dmytro is a proven Data Vault 2.0 expert and has excellent knowledge of various (cloud) architectures, data modeling, and the implementation of automation frameworks. Dmytro excels in team integration and structured project work. Dmytro has a bachelor’s degree in Finance and Financial Management.